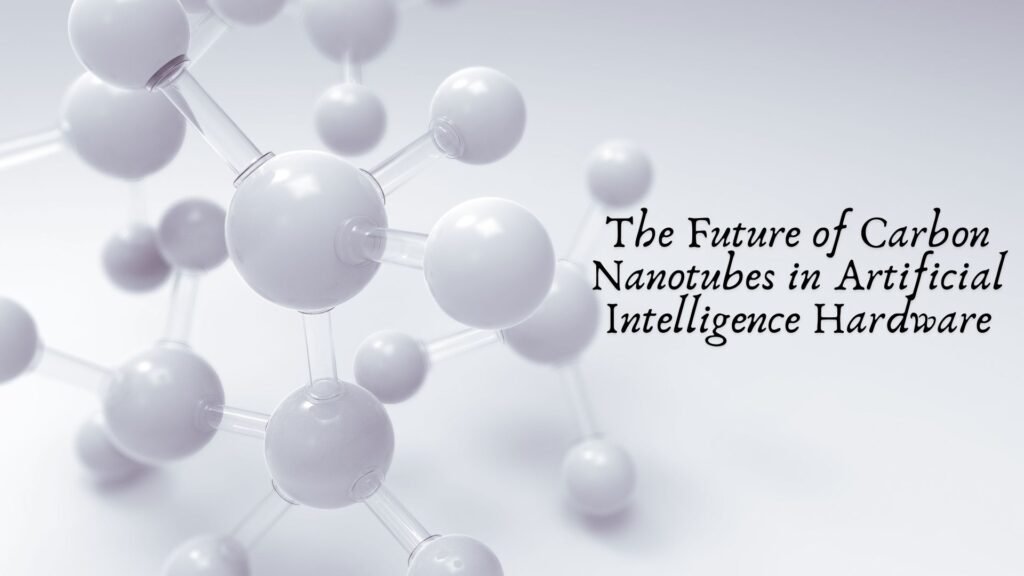
Introduction
Artificial Intelligence (AI) is rapidly transforming various industries, from healthcare to finance, by enhancing decision-making and automating complex processes. However, AI’s computational demands are growing exponentially, pushing the limits of conventional silicon-based hardware. To address these challenges, researchers are exploring alternative materials, including carbon nanotubes (CNTs). These nanomaterials have the potential to revolutionize AI hardware by providing enhanced speed, efficiency, and miniaturization. This article delves into the role of CNTs in AI hardware, their benefits, challenges, and the future prospects of this technology.
What Are Carbon Nanotubes?
Carbon nanotubes are cylindrical nanostructures composed of rolled-up sheets of graphene. They exhibit remarkable electrical, thermal, and mechanical properties, making them highly promising for electronic applications. Their unique atomic structure enables them to conduct electricity more efficiently than traditional silicon, with high electron mobility and low power dissipation. These properties make CNTs an ideal candidate for AI hardware, where speed and efficiency are critical factors.
How Carbon Nanotubes Enhance AI Hardware
The increasing complexity of AI models requires hardware capable of handling vast amounts of data with high-speed processing. CNTs offer several advantages in this regard:
Superior Electrical Properties
CNTs possess exceptional electrical conductivity, allowing them to outperform traditional silicon transistors. Their high carrier mobility enables faster signal processing, reducing computational delays and enhancing AI efficiency.
Energy Efficiency
One of the major challenges in AI hardware is excessive energy consumption. CNTs have lower resistance compared to silicon, resulting in reduced heat generation and power requirements. This can lead to more sustainable AI systems with prolonged battery life in portable devices.
Miniaturization and Density
As AI applications demand more computational power, the need for denser transistor integration grows. CNTs enable the development of ultra-small transistors, allowing for higher processing power within a smaller footprint. This is crucial for edge computing and AI-driven IoT devices.
Thermal Management
AI hardware often faces overheating issues due to prolonged high-speed computations. CNTs have excellent thermal conductivity, dissipating heat more efficiently than silicon. This reduces the risk of hardware failures and extends the lifespan of AI processors.
Current Applications of CNTs in AI Hardware
While CNT-based AI hardware is still in the experimental phase, several research initiatives and prototype developments highlight its potential:
Carbon Nanotube Transistors (CNTFETs)
CNTFETs are transistor technologies that use CNTs instead of silicon. These transistors exhibit higher switching speeds, lower power consumption, and improved efficiency. AI processors based on CNTFETs could significantly enhance machine learning model training and inference speeds.
Neuromorphic Computing
Neuromorphic chips, designed to mimic the human brain, require highly efficient materials. CNTs facilitate the creation of artificial synapses with better electrical conductivity and lower energy consumption, making them ideal for next-generation AI chips.
Memory and Data Storage
AI applications require vast memory and storage capabilities. CNT-based non-volatile memory (NVM) offers faster read/write speeds, lower power consumption, and improved durability compared to traditional flash memory.
Flexible AI Hardware
Wearable AI devices and flexible electronics benefit from CNT-based circuits due to their lightweight and flexible nature. These materials allow for the development of AI-powered smart textiles and biomedical devices.
Challenges in Implementing CNTs for AI Hardware
Despite their promising potential, several challenges must be addressed before CNT-based AI hardware becomes mainstream:
Manufacturing Scalability
Producing high-quality CNTs at a large scale with uniform properties remains a significant challenge. Current synthesis methods need refinement to achieve consistent performance across large batches of CNTs.
Integration with Existing Technology
Most AI hardware today is based on silicon technology. Transitioning to CNT-based systems requires seamless integration with existing semiconductor fabrication processes, which demands significant research and development.
Cost Considerations
While CNTs offer superior properties, their production and integration costs are currently higher than traditional silicon-based components. Economic feasibility must be improved for widespread adoption.
Material Purity and Defects
CNTs must be free from impurities and structural defects to function optimally. Controlling their quality at the nanoscale is a critical challenge that researchers are actively working to overcome.
Future Prospects of CNTs in AI Hardware
The future of CNT-based AI hardware looks promising as research and development efforts continue to progress. Several advancements could accelerate the adoption of CNTs in AI hardware:
Advances in CNT Manufacturing
New synthesis techniques, such as chemical vapor deposition (CVD) and precision placement methods, are improving CNT quality and scalability. These advancements could enable mass production of CNT-based transistors and circuits.
Hybrid AI Hardware
Combining CNT-based components with existing silicon technology could serve as a transitional step toward fully CNT-based AI processors. Hybrid architectures may offer the best of both worlds, optimizing performance while maintaining cost-effectiveness.
AI-Optimized CNT Designs
Researchers are exploring AI-driven approaches to optimize CNT transistor designs. Machine learning models can be used to predict and enhance CNT properties, leading to better hardware performance.
Quantum Computing Synergy
CNTs have potential applications in quantum computing, where their unique electrical properties could enhance qubit stability and performance. The convergence of CNT technology with quantum AI hardware could unlock unprecedented computational capabilities.
Conclusion
The future of carbon nanotubes in AI hardware is filled with exciting possibilities. Their superior electrical properties, energy efficiency, and potential for miniaturization position them as a game-changer in AI computing. However, challenges related to manufacturing, cost, and integration must be addressed before CNT-based AI hardware can become mainstream. As research advances, we may witness a new era of ultra-efficient, high-performance AI hardware driven by carbon nanotubes, paving the way for more powerful and sustainable AI applications.